What is AIOps?
Today, the systems and applications within organizations generate massive volumes of data—with some organizations experiencing millions of events per day. At this scale, it is no longer viable for humans to manually parse through all that data to detect and remediate issues. The cognitive load is worsened by the fact that organizations often have dozens of tools monitoring thousands of services—any one event that emanates from these tools may be meaningless on its own. Such phenomena have created mission-critical needs for automation, machine learning, and predictive capabilities.
AIOps, or Artificial Intelligence for IT operations, is a best practice that allows organizations to improve efficiency, resolve customer impact faster, and codify incident response processes. Essentially, AIOps solutions provide similar functionality to existing event management solutions, but add capabilities required for complex, modern environments such as machine learning, flexible data collection and ingestion, end-to-end event-driven automation, and more.
How does AIOps work?
AIOps platforms operate across data ingestion, pattern recognition, automation, and continuous learning. This process offers a holistic approach to IT operations, turning complex data into actionable insights.
- Data collection and ingestion: AIOps gathers data from multiple sources, such as server logs, network metrics, and observability platforms. Pulling in this data, AIOps offers a single pane of glass view into the health of your IT ecosystem. This process supports both structured and unstructured data, offering a comprehensive view of the IT landscape.
- Event correlation and pattern recognition: Machine learning algorithms identify patterns within the data, correlating similar events to uncover potential root causes. This process helps filter out non-essential information and prioritize the most critical alerts that require immediate action. While automation streamlines initial analysis, critical alerts are flagged for human intervention, ensuring that complex decisions and nuanced problem-solving remain in the hands of your team.
- Anomaly detection and predictive analytics: By analyzing historical trends and recognizing unusual patterns, AIOps can detect anomalies that may indicate emerging issues, enabling preemptive actions to prevent downtime.
- Automation and remediation: AIOps platforms automatically execute predefined workflows to resolve issues. For example, in a data center, an AIOps tool might detect high CPU usage and initiate a response to prevent a server overload.
- Continuous learning and feedback: As AIOps software processes data, it continuously learns from each incident, refining its predictive algorithms. This learning enhances accuracy and enables a more efficient response to similar issues in the future.
AIOps key capabilities
Some of the key capabilities of AIOps are as follows:
- Noise reduction: Organizations should be able to reduce noise across services and eliminate interruptions caused by transient alerts or alert storms. Alerts should be grouped into relevant incidents instead of kicking off a new incident each time.
- Triage and RCA: AIOps solutions should provide users with the context needed to do their jobs faster. This includes context pulled from event data and normalized, previous historical context, and current system impact.
- Automation: Organizations should be able to create and scale automation across their technological ecosystem, reducing toil and improving efficiency. This should be able to be centrally controlled as well as available self-service for individual teams.
- Visibility: AIOps solutions should be a single pane of glass that shows you your operating posture at all times, helping you answer the all-important question, “Is my system okay?”.
Let’s delve into some of the benefits of leveraging these capabilities more specifically.
Benefits of AIOps
Overall, AIOps helps teams achieve fewer incidents and faster resolution. Here are some key benefits to keep in mind:
- Easy to get started: Ideally, AIOps shouldn’t be a long, difficult implementation. And it doesn’t need to happen overnight. Most successful implementations take a staged approach. This way, you can start seeing faster resolution and fewer incidents immediately and can reclaim that time for value-add work.
- Brings teams together: AIOps isn’t just a tool for developers. It’s equally beneficial for NOCs, ITOps teams, SREs, DevOps teams, platform engineers, and everyone. All teams have something to gain from AIOps, whether that’s less noise on the front lines or the ability to craft automation across the entire technical ecosystem.
- Increases collaboration: Centralized data and insights improve cross-team communication, ensuring everyone—from developers to operations—is aligned during incidents.
- Continuously learning: AIOps should be a low-maintenance solution. But, that doesn’t mean once it’s set up that it’s complete. Machine learning (ML) is always operating in the background, learning about how your teams and organization resolve problems. It gets better with time.
- Shares next best actions: The best AIOps solutions don’t just give you data, they give you information and provide a next-best action. With AIOps, you know what to do next during an incident.
- Improves MTTR: With the right information at the right time, and incidents routed to the correct teams dynamically, organizations will see lower MTTR and therefore less customer impact.
- Speeds up MTTA: AIOps and machine learning can help to automate the decision-making process and ensure the appropriate teams are addressing the problem.
- Standardizes incident response: With normalized event data, alerts, and incidents, everyone is on the same page. And, with automation to run diagnostics and ML providing triage information previously only available in old wikis and tribal knowledge, all responders can be as effective as your best responder.
- Reduces operational costs: By automating repetitive tasks, AIOps minimizes labor costs and reduces the likelihood of expensive outages.
- Prevents burnout: With less alert noise and alert fatigue and automation acting as an L0 responder, teams can focus on the work that matters and be interrupted less, whether they’re working on the next best feature or trying to catch up on some sleep.
- Increases customer satisfaction: Faster resolutions and reduced downtime contribute to a more reliable customer experience, strengthening brand trust.
AIOps challenges
Despite its potential, there are some challenges that organizations must address for successful AIOps implementation:
- Data volume and quality: AIOps requires a significant amount of quality data. Low-quality or incomplete data can skew insights, leading to inaccurate incident detection. Organizations must prioritize data governance to ensure accurate, reliable input for AIOps systems.
- Integration with legacy systems: Older systems may lack compatibility with AIOps, hindering data collection and analysis. A phased integration plan helps organizations gradually incorporate AIOps without disrupting legacy operations.
- Scalability concerns: As organizations grow, scaling AIOps across expanded IT environments can become complex. Planning for scalability from the start, including adequate infrastructure and clear processes, helps mitigate these challenges.
- Cost of AIOps implementation: Implementing AIOps requires significant investment in both technology and training. To offset costs, organizations can prioritize high-impact areas initially, gradually scaling their AIOps capabilities.
AI in IT operations examples
AIOps has broad applications across industries, each benefiting from the technology in unique ways:
- Healthcare: Hospitals use AIOps to monitor critical systems that support patient care. When a hospital’s network experiences downtime, patient services and data access can be disrupted. With AIOps, hospitals can prevent disruptions by predicting potential system failures and automatically rerouting data to backup systems, ensuring continuous, reliable access to patient records and care systems.
- Financial services: In the financial sector, transaction speed and data security are crucial. AIOps tools help banks and financial institutions monitor network health, detect fraud patterns, and minimize downtime during peak transaction times, such as Black Friday. Predictive analytics help financial teams proactively resolve issues, ensuring smooth transactions for customers and secure systems.
- Retail: Retailers experience high traffic during sales and holiday seasons, often leading to system overloads. AIOps enables real-time monitoring and quick incident response, ensuring consistent service availability and an uninterrupted shopping experience for customers. By automating responses to remove performance bottlenecks, retailers can ensure smooth operations even during peak demand periods.
AIOps Use Cases
AIOps can be a game-changer in a variety of use cases:
- Networking Operation Center (NOC) modernization: For NOCs, AIOps centralize monitoring and automate initial diagnostics, allowing teams to focus on high-priority events and reduce alert fatigue. AIOps act as the single source of truth, providing complete visibility across the IT infrastructure and helping teams transition from reactive to proactive responses.
- Major Incident Management (MIM): AIOps can help organizations quickly detect major incidents. And, with the right context via ML, triage information and historical context gives these teams a leg up in the moments that matter most.
- Distributed service owners: Service owners have the right amount of autonomy and are able to create their own automation and noise reduction criteria to ensure that they, as the subject matter experts (SMEs), are pulled away from value-add work only when necessary.
- Incident response and root cause analysis: AIOps rapidly identifies incidents and uses ML-based root cause analysis to determine the underlying issue. Auto-remediation can also enable the platform to automatically resolve specific types of incidents or initiate corrective actions. For example, if an AIOps platform detects a recurring server issue, it could automatically trigger a corrective script or perform preventative maintenance, reducing time to resolve (TTR) and the frequency of future incidents.
- Compliance and security: AIOps can monitor for security breaches and unusual activity, identifying potential threats. By automatically flagging these issues and initiating a response, AIOps helps organizations maintain records to help with compliance and strengthen data security.
Future trends in AIOPs
As AIOps evolves, its potential to enhance development and IT operations processes grows, addressing pain points like incident management, real-time anomaly detection, and automation of repetitive tasks. Here are the key trends developers and engineers should keep an eye on as AIOps continues to advance.
1. Hyperautomation for IT workflows
Hyperautomation in AIOps leverages a mix of RPA, low-code/no-code platforms, and AI-driven automation to streamline complex workflows. For developers and engineers, this trend means automating not just individual processes but entire workflows across the DevOps pipeline.
Imagine an automated pipeline where AIOps handles everything from triggering builds, running tests, deploying code, monitoring performance, and rolling back changes if anomalies arise. By linking AIOps with CI/CD tools, engineering teams can achieve continuous integration and deployment without needing to manually intervene in each stage, freeing up time to focus on innovation and new feature development.
2. AI-driven decision-making for incident response
Future AIOps platforms won’t just flag issues; they’ll make autonomous decisions based on incident patterns, resource impacts, and previous resolution effectiveness. This trend is particularly relevant for developers and engineers who often juggle real-time troubleshooting with ongoing development tasks.
Reinforcement learning models will refine incident response paths over time, allowing AIOps to automatically escalate or resolve issues based on incident severity and historical outcomes. For example, if a specific memory leak has been resolved by rebooting the server in the past, the AIOps system might automatically execute this solution. By automating these decisions, developers are less likely to be pulled into firefighting, keeping them focused on productive development efforts.
3. Seamless integration with edge computing and IoT
With the rise of edge computing and IoT, managing data at scale requires high responsiveness and reduced latency. AIOps will expand its capabilities to support real-time monitoring and incident management across distributed devices and environments, which is essential for engineering teams working with IoT or distributed systems.
For instance, in an IoT setup with thousands of connected devices, AIOps can identify and address latency or connectivity issues directly at the edge, triggering responses to prevent data loss or performance dips. Engineers developing applications for IoT can leverage AIOps to ensure device reliability and system uptime, even when systems are highly decentralized.
4. Customizable solutions for specific engineering environments
As AIOps platforms mature, vendors are expected to offer more customizable options, including templates and pre-trained models that align with specific engineering needs. This will be especially valuable for software teams in sectors like fintech, healthcare, and telecom, where compliance and uptime requirements differ.
For example, developers in finance could use AIOps to prioritize compliance, configuring the platform to detect and escalate anomalies in transaction logs. In a telecom context, AIOps could be fine-tuned to monitor network health and service performance. This ability to tailor AIOps configurations to industry-specific requirements will help engineers address technical debt and compliance with less manual effort.
5. Conversational AIOps using Natural Language Processing (NLP)
As Natural Language Processing (NLP) improves, conversational interfaces for AIOps are gaining traction, offering developers and engineers a more intuitive way to interact with AIOps systems. By integrating NLP, AIOps will allow engineers to query systems directly, speeding up information retrieval.
Consider a scenario where a developer wants to quickly understand the status of a deployment: they might ask, “What’s the current status of the production environment?” or “List recent incidents and resolutions.” AIOps with NLP capabilities can provide this information without requiring deep-dive searches into logs, reducing context-switching and making troubleshooting more efficient.
6. Autonomous IT operations
Autonomous IT operations represent a major leap forward, where systems are self-managing and self-healing. For developers and engineers, this trend reduces the need for on-call firefighting and creates more time for strategic engineering tasks.
An autonomous AIOps platform might monitor application health in real-time, auto-scaling resources during traffic spikes, or initiate rollbacks for deployments with high error rates. Engineers can rely on these self-healing capabilities to support continuous availability, reducing the need for 24/7 manual oversight and improving service reliability.
7. Enhanced threat detection and proactive security
AIOps platforms will continue to advance in cybersecurity, integrating with Security Information and Event Management (SIEM) and Security Orchestration, Automation, and Response (SOAR) systems to identify threats and automate responses. For engineers, this trend enables proactive security, essential for protecting applications and user data in cloud-native and microservices architectures.
For instance, an AIOps system could monitor network traffic for suspicious patterns, immediately flagging and isolating a compromised server while alerting security teams. Developers and engineers working on applications that handle sensitive information can rely on AIOps to enforce security measures automatically, ensuring compliance and reducing vulnerabilities without adding manual checks.
8. Self-optimizing systems and continuous feedback loops
Self-optimization in AIOps is gaining importance as systems become more complex and dynamic. Future AIOps platforms will use feedback loops to continuously fine-tune their algorithms, adapt to new patterns, and adjust their responses based on real-world outcomes.
For developers and engineers, this means AIOps can dynamically adjust alert thresholds and correlation rules, refining their own processes without human intervention. If a certain threshold consistently triggers false positives, the AIOps system might lower its sensitivity in that area, ensuring that engineering teams only get alerts for meaningful events. This adaptive capability allows AIOps systems to “learn” and reduce the volume of low-priority notifications, making them more valuable as they evolve.
9. Correlating IT metrics with business and user impact
As AIOps grows in sophistication, it will become more adept at correlating technical metrics with business outcomes, helping engineering teams understand how their work impacts user experience and revenue. By correlating metrics like page load time, API response time, or server availability with user satisfaction and sales metrics, AIOps platforms will provide valuable insights into the real-world effects of technical performance.
For instance, if an API latency issue is impacting the checkout process on an e-commerce site, AIOps can identify the relationship between latency and cart abandonment rates, alerting engineers to prioritize this fix. This trend helps developers align their work with broader business objectives, ensuring that engineering decisions are informed by end-user impact.
Build vs buy: How to get the most out of AIOps
Choosing between building a custom AIOps platform or purchasing a commercial solution depends on the organization’s specific needs, resources, and goals.
- Building a custom solution: Building a custom AIOps solution offers complete control but requires substantial resources. Custom solutions are highly flexible but may be time-intensive to develop and maintain, and they risk becoming outdated as technology advances.
- Purchasing a pre-built solution: Commercial AIOps platforms are designed with best practices in mind, offering robust features, scalability, and regular updates. These platforms integrate with existing systems, reducing the complexity and cost of implementation. Many vendors also offer customer support, ensuring smooth deployment and adoption.
For optimal results with AIOps, organizations should focus on best practices that align with long-term operational goals:
- Define clear metrics and objectives: Identify the key metrics for AIOps success, such as MTTR reduction, operational cost savings, or improved incident response rates. These metrics help track AIOps performance over time.
- Start with pilot projects: Testing AIOps in specific areas allows teams to understand its impact and refine processes before scaling. For example, using AIOps initially for incident management offers a low-risk, high-impact starting point.
- Encourage cross-functional adoption: AIOps works best when adopted across departments. Encourage collaboration between IT, DevOps, and business teams to ensure the solution is tailored to diverse operational needs.
- Optimize feedback loops: Use feedback loops to improve AIOps algorithms. Regularly reviewing performance helps the platform learn from each incident, refining predictive analytics and automated responses.
- Invest in continuous training: Ongoing training is essential for teams to maximize the potential of AIOps tools. By staying updated on new features and techniques, teams can keep up with evolving AIOps capabilities.
PagerDuty AIOps helps teams achieve fewer incidents and faster resolution with no maintenance required and no length implementations. To learn more about PagerDuty AIOps, you can watch this short on-demand webinar.
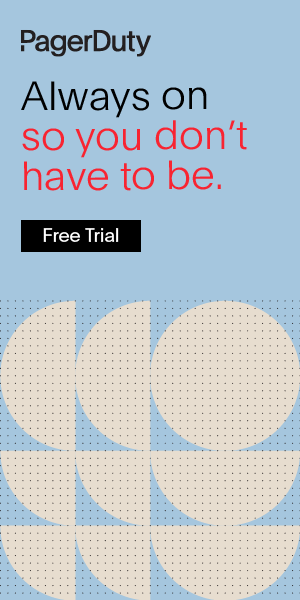
Additional
Resources
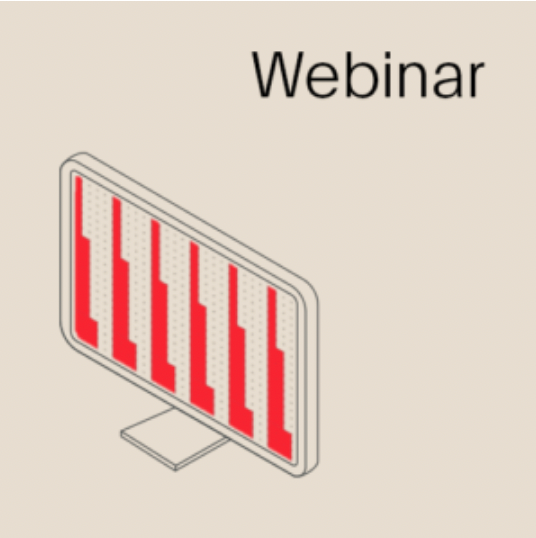
Webinar
Leveraging LLMs to Deliver Excellence in IT Operations
Whitepaper
ServiceOps Executive Playbook Modernizing IT Service Management